The rebound: How Covid-19 could lead to worse traffic (v2)
A rush to single occupancy vehicles could result in large travel time increases in transit heavy communities.
By: Yue Hu, Will Barbour, Samitha Samaranayake, Dan Work
Update 05/25/2020: The Rebound has been updated with new models that account for prediction uncertainty. The prior version of the article has been archived at The Rebound v1.
Update 07/20/2020: A label bug was fixed in the Rebound Calculator where the total travel time was reported in the data table.
Rebound Calculator
The findings of The Rebound study are coalesced into the Rebound Calculator. The tool estimates one-way commute travel times using models built around recent commuting data for most U.S. metro areas. As the number of vehicles on the roads increases, so too does the travel time, according to a traffic fundamental called the BPR model. Travel times, therefore, increase if existing commuters switch from transit or carpool to single-occupancy vehicles. Travel times will decrease if fewer vehicles are on the road due to unemployment or remote work. How mode shift could affect travel times is particularly important in the era or Covid-19, as it could impart high cost on commuters due to increased time spent in traffic.
Select a city using the drop down menu in the calculator and select mode shift values using the sliders. The plots show predicted one-way travel times and mode share. The table shows the added one-way travel time with uncertainty, plus the added round-trip cost of congestion per driver per year and added cost of congestion across the metro area per day [1]. Models are based on data from the census-defined metropolitan statistical areas, which include areas outside a city’s urban core. Note that these one-way travel time predictions use a baseline of 2018 commute data from the American Community Survey; they are not corrected for 2020 pre-Covid conditions.
The sliders in the calculator change values in the following way:
- Transit shift to SOV: This is the percent of transit commuters who switch to single occupancy vehicles. At 0%, all transit users in the pre-covid setting return to transit. Setting this number to 25% means one in four transit users switch to single occupancy vehicle trips instead. Each transit commuter who switches to a single occupancy vehicle adds a vehicle to the road, increasing travel times. Increasing this number will increase travel times.
- Carpool shift to SOV: Similar to transit shift to SOV, this is the percent of carpool commuters who switch to single occupancy vehicles. Increasing this number will increase travel times.
- Jobs lost (%): This is the percent of commuters who no longer travel to work because they are unemployed. For example, setting this at 10% reduces the number of single occupancy vehicle trips by 10%, the number of transit riders by 10%, and so on. The job loss rates are assumed to be evenly lost across the traveling modes. Increasing this number will reduce travel times.
- Shift to remote work: This is the percent of commuters who no longer travel to work because they are working from home. Like the jobs lost slider, setting this slider at 10% reduces the number of travelers by 10%, with the 10% reduction occurring for each mode. Increasing this number will reduce travel times.
For more details about how the calculator was created, see the remainder of the article below.
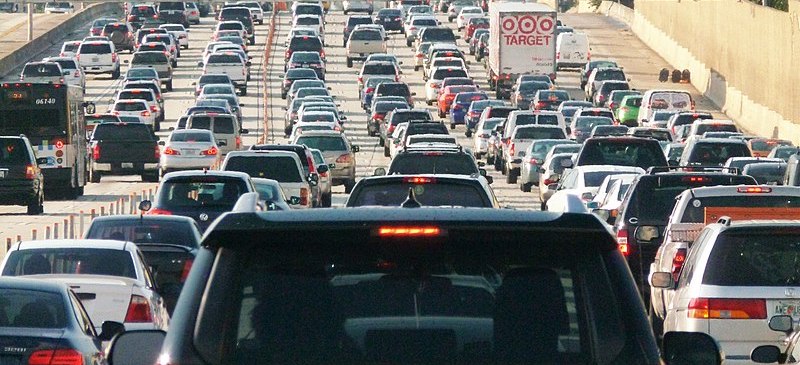
Summary findings
Traffic jams 101. Traffic jams result when there are more vehicles than the road network can handle. After the roads are fully saturated, the travel times increase with each additional traveler. In fact, the rate at which traffic grows follows a very predictable pattern.
Similarly, when there is a steep decrease in the number of travelers, traffic can vanish. The travel restrictions put in place to reduce the spread of Covid-19 resulted in a sharp reduction in traffic throughout the US.
As communities begin to reopen, it is important to understand how quickly traffic will rebound. Using basic laws of traffic, we can predict the amount of traffic that will occur in each city given only the number of vehicles on the road. This allows us to explore “what if” scenarios. For example, it is possible that many transit riders could switch permanently or semi-permanently to a car, instead. We want to know how these decisions will impact traffic on our roadways.
Main point. Cities that depend on transit are at risk for extreme traffic unless transit systems can resume safe, high throughput operations quickly. For a 25% mode shift from transit and carpool to SOV, the cities most at risk include:
- San Francisco: additional 16.4 minutes (±3.2 minutes) round trip commute travel time, +16.4m per day congestion cost.
- New York: +11.4 min (±4.2 min) round trip, +$42.0m/day congestion cost.
- Los Angeles: +1.8 min (±1.0 min) round trip, +$6.0m/day congestion cost.
- Boston: +4.4 min (±1.6 min) round trip, +$5.2m/day congestion cost.
- Chicago: +2.0 min (±1.0 min) round trip, +$4.4m/day congestion cost.
- Seattle: +4.4 min (±1.0 min) round trip, +$4.2m/day congestion cost.
- San Jose: +3.8 min (±2.8 min) round trip, +$2.0m/day congestion cost.
These potential increases are avoidable. If transit ridership returns in step with trips by car, road traffic will return to normal. Monitoring closely road and transit ridership during the rebound is critical.
In the remainder of this article, we will:
- Introduce the data on traffic in US cities
- Explain how to model traffic trends in these cities
- Use the model to forecast traffic in different reopening scenarios
Data analysis
In order to understand traffic in cities, we explore travel data from the American Community Survey (ACS) from the United States Census Bureau (USCB). Using this data, we can answer questions like:
- How many cars are on the road in each city?
- What is the average travel time [2] experienced by commuters in each city?
This publically available data is reported annually, and most metropolitan statistical areas have data from 2010-2018 [3]. We automatically downloaded it via Julien Leider’s "censusdata" Python package.
Determining the number of cars on the road is a straightforward calculation. The American Community Survey details the number of commuters in single occupancy vehicles (i.e., 1 car per person); the number of 2-person carpools, 3-person carpools, etc.
Once the number of vehicles are determined, we can begin to compare cities. The plot below shows the one-way travel time and the number of vehicles for most major cities in the US in 2018, which is the most recent data that is available.
Not surprisingly, cities with a large number of vehicles tend to have higher travel times. But the trends are not obvious, since the travel time in each city depends on a number of factors, such as the size of the city, the population density, the average distance traveled, etc.
To better understand the travel times, we can look at the behavior of individual cities. It turns out that for many cities, travel times follow a regular pattern known as the BPR model. The BPR model was originally designed to relate the travel times on a single road, but can also be applied to cities. The important point about the model is it captures common sense. When the roads are not completely packed, travel times are low. But after the number of cars is larger than a certain amount, travel times can skyrocket.
When applied to each city, the BPR model allows us to estimate two important numbers:
- Empty-road travel time: This is the average travel time commuters experience if the roads are virtually empty and free of traffic.
- Road capacity: This is the number of cars the city can handle before travel times quickly rise.
The BPR model was fit to each city using Bayesian Ridge Regression. Importantly, this technique allows us to estimate a confidence interval for the prediction, which is reflected in the calculator.
Once we know the empty road travel time and the road capacity for each city, we can standardize each city and compare them again. Instead of plotting travel times against the number of vehicles, we will instead look at:
- Travel time ratio: A travel time ratio of 1.1 means the current travel time is 1.1 times (or 10% higher) than the empty road travel time. Similarly, a travel time ratio of 1.5 means the current travel time is 1.5 times (50% higher) then the empty road travel time.
- Capacity ratio: A capacity ratio of 1.1 means there are 10% more cars than the road capacity. A capacity ratio of 0.9 means there are 10% fewer cars than the road capacity, and so on.
When we plot the cities and compare the travel time ratios with the capacity ratio, we see that all of the cities follow the same growing curve. This is the same data from Figure 1 above, just standardized to account for the fact that larger cities tend to have larger capacities and longer commutes, even when the roads are empty.
The fact that the cities fall on a nice curve means traffic is predictable [4].
Traffic scenarios during the rebound
The Rebound Calculator introduces the ability to set a customized traffic and mode share scenario, consisting of four factors: transit shift to SOV, carpool shift to SOV, job loss, and remote work. Each factor is set relative to its baseline 2018 level. Transit and carpool shift use 2018 mode share as a baseline, and job loss and remote work are in terms of 2018 commuters taken off the road by each factor.
We report, for a given scenario, the added travel time (relative to 2018 baseline) and the one standard deviation uncertainty of this prediction. The additional cost of congestion is given in terms of dollars per driver per year, and in terms of dollars per metro area per year. The cost of congestion is measured in additional hours spent traveling, relative to the 2018 baseline. Each added hour is valued at $18.58, the 2018 national median hourly wage as reported by the Bureau of Labor Statistics.
Generally, we see that the travel time increases are more severe for larger cities, and those that are more dependent on transit -- 8 of 10 cities with the largest predicted travel time increase also rank in the top 10 for transit use. More extreme scenarios, such as 50+% of transit or carpool riders shifting to SOV are extremely unlikely. But it does illustrate that keeping transit open, safe, and available will be important to control the traffic rebound.
In less extreme scenarios (e.g., 10-25% mode shift), we see that average travel times can increase by a few minutes in some metro areas. This might not seem like much, but it is. The additional delay is for each commute trip taken. When multiplied by the number of travelers in these cities, even a minute of delay per traveler can easily result in thousands of hours of additional time spent in traffic every day, costing residents millions of dollars in lost time.
A few take home messages
- When the roads are already saturated, adding cars is detrimental to everyone’s commute. This is a basic law of traffic.
- Covid-19 cleared the streets in many communities. As we reopen, traffic will eventually rebound. If transit ridership does not return, travel times will increase, sometimes dramatically.
- Travel time increases of 5-10 minutes are possible in high-transit cities, which adds up to hundreds of thousands of hours of additional travel time each day and millions of dollars in lost time.
- These increases are avoidable, if transit ridership resumes in step with car traffic. Investing money in safe transit during Covid-19 conditions can save money overall, compared to the alternative cost of increased travel times.
Notes
[1]Cost of congestion is measured by additional hours spent in traffic, relative to 2018. Each added hour is valued at $18.58, the 2018 national median hourly wage as reported by the Bureau of Labor Statistics. This measure is used in different variants by other studies on congestion such as the Texas A&M Urban Mobility Report.
[2]The travel times are average over the population and the day. This means people traveling during rush hours have longer commutes, and people traveling off peak have shorter ones.
[3]The data is from 2018. It is the most current data we have. The travel times were probably higher in 2019.
[4]Many, but not all cities follow the law! Some cities do not have enough variation in the census data for us to accurately determine how they behave. More details on methods and technical approaches coming next.
Research team (contact us)
- Yue Hu is a PhD student at Vanderbilt University
- Will Barbour is a research scientist at Vanderbilt University
- Samitha Samaranayake is an assistant professor at Cornell University
- Dan Work is an associate professor at Vanderbilt University